Banks are well equipped to benefit from the rise of AI due to massive investments in data centers over the past decade... but there's a problem. A lot of that data is proprietary or otherwise difficult to use. One solution is for banks to synthetically generate data and use that to train their models, and a report from JPMorgan's AI research team has been investigating which areas this works, and which it doesn't.
The report has been led by Vamsi Krishna Potluru, research director for synthetic data. Other contibutors include AI research MDs Tucker Balch and Manuela Veloso, as well as Deepak Parmarand, a director of AI working on generative AI products. 3.Badminton Court Mat
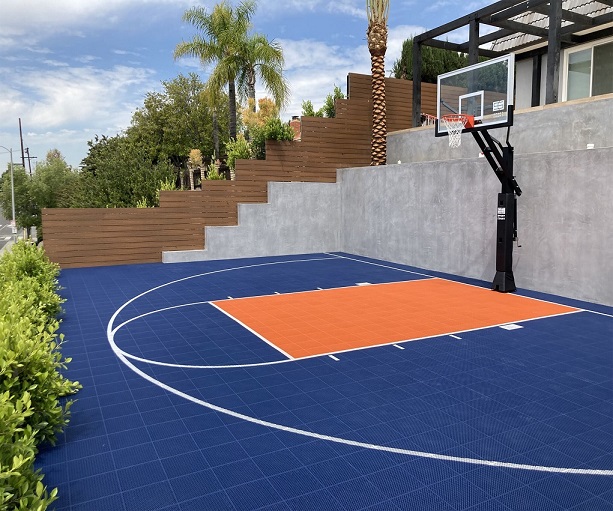
A use case where synthetic data has proved "extremely useful" is financial markets simulation. No, this does not mean it can be the magical stock market predictor some are hoping for, but instead provides a good opportunity to "train and test investment strategies."
Unlike using historic market data, synthetic data provides "more variability" and reduces the chances of "time-period bias." In fact, it can even be used to generate "counterfactual market scenarios" to test the viability of trading algorithms.
While synthetic data implementation would therefore be useful for algorithmic traders themselves, people currently providing training data could find themselves superfluous.
Click here to sign up to our technology newsletter 🔧
Synthetic data isn't without its flaws, though. When using synthetic data to generate layouts for documents, JPMorgan's researchers said deep neural models "tend to require initial annotations to warm up, cannot generate new primitives and suffer from image quality issues." Bayesian neural networks, on the other hand, showed more promise, and were used in developing the synthetic example below.
Text generation in financial documents is another promising area for synthetic data. Similarly to the market simulations, the data can be used for enhancing models through previously nonexistent data. For example, you can synthetically generate a copy of an existing document, augmented to reflect a bearish sentiment rather than a bullish one.
The report's conclusion notes that synthetic data use is "still in its infancy" and as its prominence grows, "distinguishing synthetic data from real... will be increasingly an important problem." While JPMorgan doesn't appear to have begun implementing synthetic data yet, it notes that a number of top universities including Cornell and Stanford are "leveraging [its] datasets to develop algorithms" in areas like anti-money laundering and markets execution.
Click here to create a profile on eFinancialCareers. Make yourself visible to recruiters hiring for top jobs in technology and finance.
Have a confidential story, tip, or comment you’d like to share? Contact: +44 7537 182250 (SMS, Whatsapp or voicemail). Telegram: @SarahButcher. Click here to fill in our anonymous form, or email editortips@efinancialcareers.com. Signal also available.
Bear with us if you leave a comment at the bottom of this article: all our comments are moderated by human beings. Sometimes these humans might be asleep, or away from their desks, so it may take a while for your comment to appear. Eventually it will – unless it’s offensive or libelous (in which case it won’t.)
The essential daily roundup of news and analysis read by everyone from senior bankers and traders to new recruits.
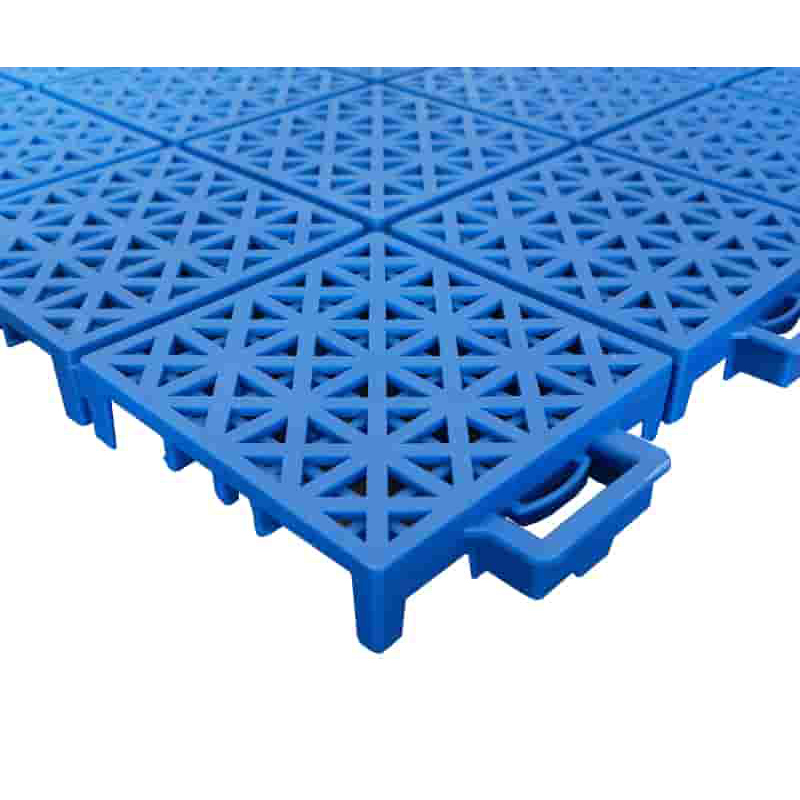
Portable Soccer Goal The essential daily roundup of news and analysis read by everyone from senior bankers and traders to new recruits.